Table Of Content

While this algorithm is fairly straightforward, it is also quite tedious and is limited to 2n factorial designs. Thus, modern technology has allowed for this analysis to be done using statistical software programs through regression. The interaction effects situation is the last outcome that can be detected using factorial design. From the example above, suppose you find that 20 year olds will suffer from seizures 10% of the time when given a 5 mg CureAll pill, while 20 year olds will suffer 25% of the time when given a 10 mg CureAll pill. When 40 year olds, however, are given a 5 mg pill or a 10 mg pill, 15% suffer from seizures at both of these dosages.
Informal introduction to factorial experimental designs
This can be conducted with or without replication, depending on its intended purpose and available resources. It will provide the effects of the three independent variables on the dependent variable and possible interactions. The use of a control group is an important experimental design method that involves having a group of participants that do not receive the treatment or intervention being studied. The control group is used as a baseline to compare the effects of the treatment group. In other words, sunlight and watering frequency do not affect plant growth independently.
Selecting the Right Factors and Components in a Factorial Design: Design and Clinical Considerations
A design which manipulates one independent variable between subjects and another within subjects. As with any statistical experiment, the experimental runs in a factorial experiment should be randomized to reduce the impact that bias could have on the experimental results. This framework can be generalized to, e.g., designing three replicates for three level factors, etc.
Types of Factorial Designs
In a simple within-subjects design, each participant is tested in all conditions. In a factorial experiment, the decision to take the between-subjects or within-subjects approach must be made separately for each independent variable. In a between-subjects factorial design, all of the independent variables are manipulated between subjects. For example, all participants could be tested either while using a cell phone or while not using a cell phone and either during the day or during the night. This would mean that each participant would be tested in one and only one condition. In a within-subjects factorial design, all of the independent variables are manipulated within subjects.
Definition of 3D Printing Parameters by the Design of Experiments to Characterise Carbon Fibre-Reinforced Polyamide
These measures may be invasive or non-invasive, and may be administered in a laboratory or clinical setting. This involves dividing participants into subgroups or blocks based on specific characteristics, such as age or gender, in order to reduce the risk of confounding variables. Blinding involves keeping participants, researchers, or both unaware of which treatment group participants are in, in order to reduce the risk of bias in the results. This involves randomly assigning participants to different groups or treatments to ensure that any observed differences between groups are due to the treatment and not to other factors. Laboratory experiments are conducted under controlled conditions, which allows for greater precision and accuracy.
Advances, challenges and opportunities in creating data for trustworthy AI
Factorial design is an important method to determine the effects of multiple variables on a response. Traditionally, experiments are designed to determine the effect of ONE variable upon ONE response. Fisher showed that there are advantages by combining the study of multiple variables in the same factorial experiment. Factorial design can reduce the number of experiments one has to perform by studying multiple factors simultaneously. Additionally, it can be used to find both main effects (from each independent factor) and interaction effects (when both factors must be used to explain the outcome).
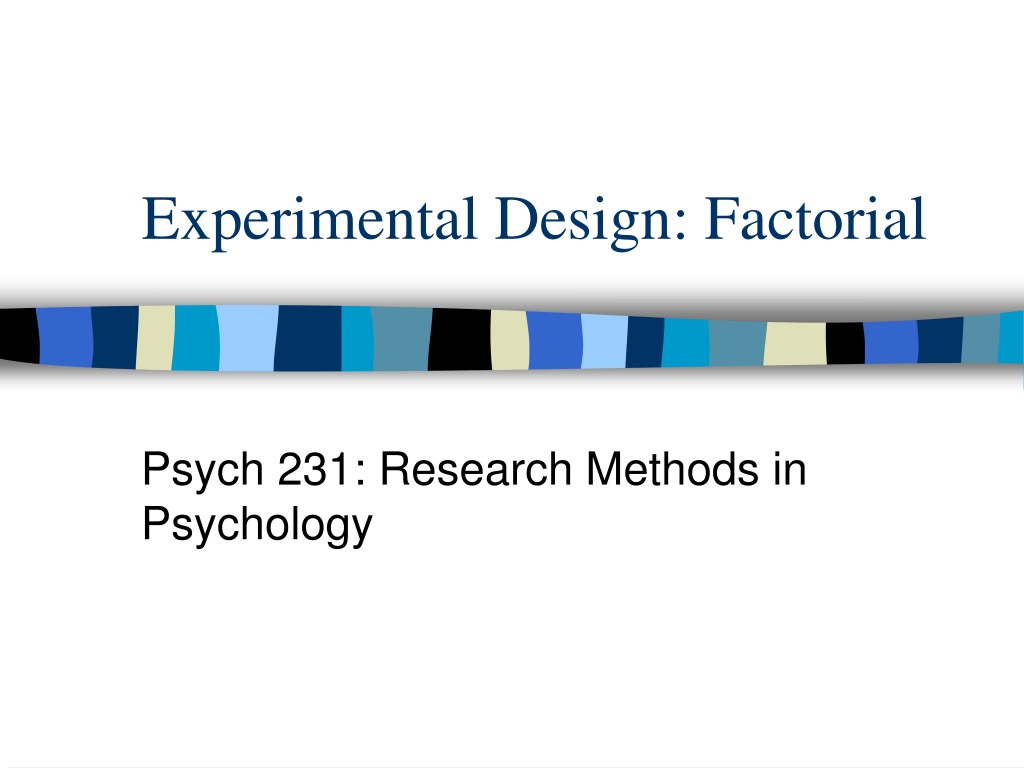
In this regard, “off” conditions (connoting a no-treatment control condition as one level of a factor) have certain advantages. They are relatively easy to implement, they do not add burden to the participants, and they should maximize sensitivity to experimental effects (versus a low-treatment control). Of course, less intensive (versus no-treatment) control conditions might be used for substantive reasons or because they ensure that every participant gets at least some treatment. Shows how each level of one independent variable is combined with each level of the others to produce all possible combinations in a factorial design. If these values represent "low" and "high" settings of a treatment, then it is natural to have 1 represent "high", whether using 0 and 1 or −1 and 1.
What is a Factorial Experiment?
This website is using a security service to protect itself from online attacks. There are several actions that could trigger this block including submitting a certain word or phrase, a SQL command or malformed data. Introduction to Statistics is our premier online video course that teaches you all of the topics covered in introductory statistics. Statology Study is the ultimate online statistics study guide that helps you study and practice all of the core concepts taught in any elementary statistics course and makes your life so much easier as a student.
We believe that their potential to yield unique data, and to do so efficiently, should make factorial screening experiments a core strategy in the process of developing effective treatments (Collins et al., 2016). For instance, not only do such designs permit the screening of multiple intervention components in a single experiment, but compared with RCT designs, factorial experiments permit more precise estimates of mediational effects. This paper highlights decisions and challenges related to the use of factorial designs, with the expectation that their careful consideration will improve the design, implementation, and interpretation of factorial experiments. Chakraborty et al., (Chakraborty et al., 2009) noted that factorial designs may not perform optimally for intervention selection in cases where there are weak main effects, but relatively strong interaction effects. Unfortunately, this situation may be a fairly common occurrence in factorial experiments of clinical interventions (e.g., Cook et al., 2016; Piper et al., 2016; Schlam et al., 2016).
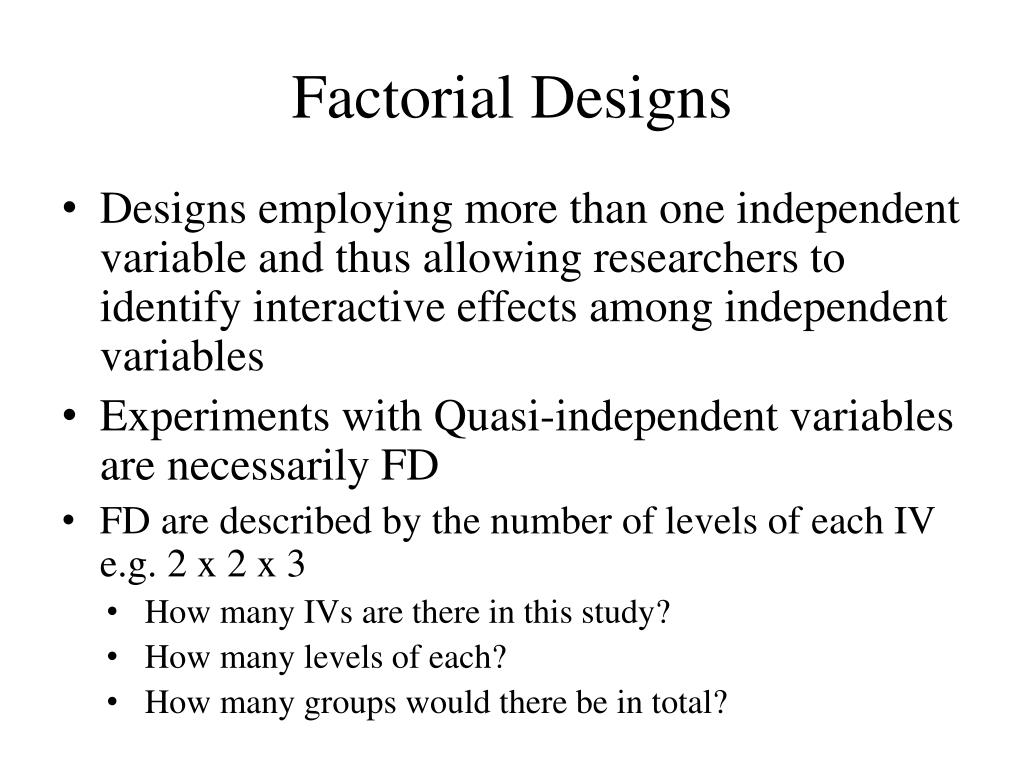
Recall that in a between-subjects single factor design, each participant is tested in only one condition. In a between-subjects factorial design, all of the independent variables are manipulated between subjects. For example, all participants could be tested either while using a cell phone or while not using a cell phone and either during the day or during the night. Such studies are extremely common, and there are several points worth making about them. First, non-manipulated independent variables are usually participant variables (private body consciousness, hypochondriasis, self-esteem, gender, and so on), and as such, they are by definition between-subjects factors.
There are three main types of factorial designs, namely “Within Subject Factorial Design”, “Between Subject Factorial Design”, and “Mixed Factorial Design”. Computerized measures involve using software or computer programs to collect data on participants’ behavior or responses. These measures may include reaction time tasks, cognitive tests, or other types of computer-based assessments. However, we can also perform a two-way ANOVA to formally test whether or not the independent variables have a statistically significant relationship with the dependent variable. Plotting the means is a visualize way to inspect the effects that the independent variables have on the dependent variable. Consequently, this procedure offers a more efficient and cost-effective means to test several combinations of two or more conditions at the same time.
Changes in worker productivity can be reasoned, for example, to be influenced by salary and other conditions, such as skill level. One way to test this hypothesis is by categorizing salary into three levels (low, moderate, and high) and skills sets into two levels (entry level vs. experienced). The challenges that may arise in interpreting interactions support strategies to select ICs based chiefly on main effects (Collins et al., 2009; Wu & Hamada, 2011), which is the approach taken in engineering and other fields that use screening experiments (Wu & Hamada, 2011). For instance, relative to some complex interactions, main effects are more easily interpreted (Collins et al., 2014); a factor’s main effects are interpretable even when it interacts with other factors. When effect coding is used, each effect is orthogonal to every other effect in the analysis model (orthogonal when the n’s are equal in each experimental condition, and nearly orthogonal when the n’s differ by a modest amount). Thus, a significant main effect reflects an experimental effect that occurs on average across all other factors in the model even when the relevant factor is involved in significant interactions (Chakraborty et al., 2009).
Utilisation of Design of Experiments Approach to Optimise Supercritical Fluid Extraction of Medicinal Cannabis ... - Nature.com
Utilisation of Design of Experiments Approach to Optimise Supercritical Fluid Extraction of Medicinal Cannabis ....
Posted: Thu, 04 Jun 2020 07:00:00 GMT [source]
You can always spot an interaction in the graphs because when there are lines that are not parallel an interaction is present. If you observe the main effect graphs above, you will notice that all of the lines within a graph are parallel. In contrast, for interaction effect graphs, you will see that the lines are not parallel. When the factors are continuous, two-level factorial designs assume that the effects are linear.
The alpha value, which determines the limit of statistical significance, can be chosen in this menu also. A common one to select is "Residuals versus fits" which shows how the variance between the predicted values from the model and the actual values. The above table contains all the conditions required for a full factorial DOE. Minitab displays the standard order and randomized run order in columns C1 and C2, respectively.
No comments:
Post a Comment